Understanding Diffusion Models: A Unified Perspective는 3가지 포스트로 나누어져 있습니다. 이전 포스팅을 참고해주세요.
Paper: https://arxiv.org/abs/2208.11970
Understanding Diffusion Models: A Unified Perspective
Diffusion models have shown incredible capabilities as generative models; indeed, they power the current state-of-the-art models on text-conditioned image generation such as Imagen and DALL-E 2. In this work we review, demystify, and unify the understandin
arxiv.org
1. ELBO, VAE, HVAE
https://donghyun99.tistory.com/14
[논문 리뷰] Understanding Diffusion Models: A Unified Perspective (1)
Understanding Diffusion Models: A Unified Perspective는 Google Brain에서 Diffusion model에 대한 이해를 돕기위해 만든 논문입니다. 이번 기회에 Diffusion model을 이해하기 위한 여러 수식이나 정의를 정리해 보려 합
donghyun99.tistory.com
2. Variational Diffusion Models
https://donghyun99.tistory.com/16
[논문 리뷰] Understanding Diffusion Models: A Unified Perspective (2)
Understanding Diffusion Models: A Unified Perspective는 3가지 포스트로 나누어져 있습니다. 이전 포스팅을 참고해주세요. Understanding Diffusion Models: A Unified Perspective (1) Understanding Diffusion Models: A Unified Perspective
donghyun99.tistory.com
Score-based Generative Models
지난번 포스트에서는 VDM이 neural network인 sθ(xt,t)를 통해 score function인 ∇logp(xt)를 심플하게 예측할 수 있다는 것을 보여주었습니다. 다만 이것은 Tweedie's Formula를 통해 예측한 값을 대입해준 것일 뿐 score function에 대한 정확한 insight가 없습니다.
따라서 이번에는 Score-based Generative Model을 통해 score function이 어떤 식으로 계산되는지를 알아보겠습니다. 결론적으로 보면 VDM이 유도하는 수식과 동일하지만 해석의 방향성으 조금 다릅니다.
Energy-based Models
Score-based generative Models을 알기 위해서는 Energy-based Models에 대한 이해가 필요합니다. Energy-based Models은 Yann LeCun이 자세하게 설명한 ppt가 있습니다.
http://www.cs.toronto.edu/~vnair/ciar/lecun1.pdf
내용을 요약해보겠습니다.
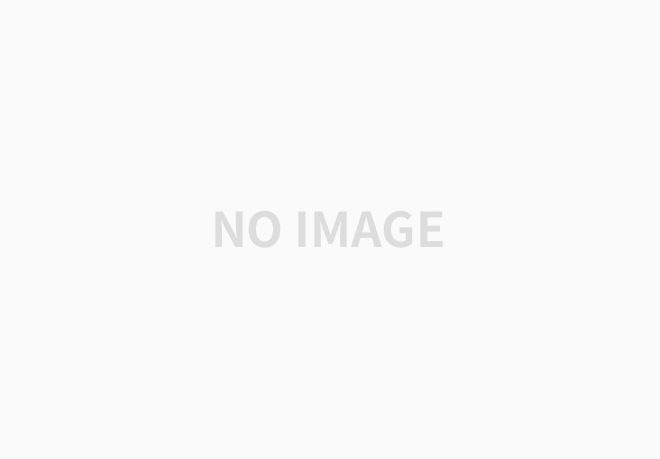
위의 그림은 이미지에 대한 Classification을 하는 Task입니다. 즉 우리는 X에 대한 알맞는 Y를 찾아내는 것을 목표로 합니다. Energy는 X와 Y를 어떠한 공간에 대한 좌표 축으로 봤을 때, 각 샘플들이 매핑되는 하나의 Scalar 값을 의미합니다.
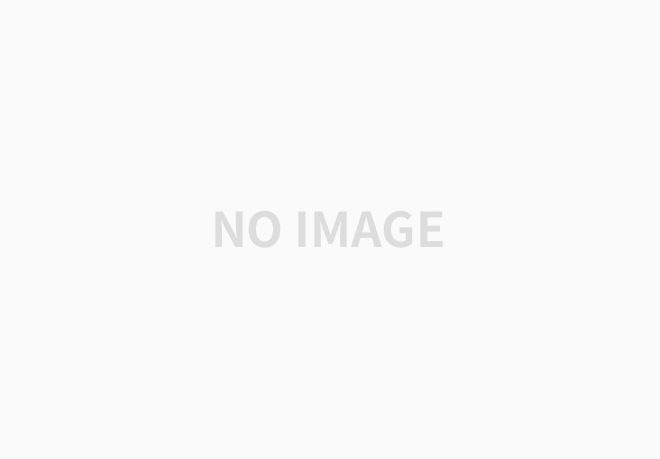
이때 Energy Function은 데이터 분포가 큰 곳은 Energy가 더 적고, 반대로 분포가 적은 곳에 대해서는 Energy가 더 커지도록 에너지를 생성하는 것을 학습합니다. Supervised 기반 학습에서는, 어떻게 보면 Loss 함수의 값과 비슷합니다. 데이터 분포가 몰려있는 쪽의 Loss가 더 낮으니까요. (저는 그렇게 이해했습니다.)
Energy-Based GAN(EBGAN)은 Discriminator를 Energy Function으로 보고 높은 분포의 입력은 낮은 값을, 낮은 분포의 입력은 높은 값을 출력하도록 모델을 구성했습니다. 단순히 real/fake를 1,0으로 분류하는 기존의 모델들과는 차별점이 있는 방식이였죠. Discriminator를 Auto Encoder로 만들어 입력 이미지를 복원시키고, 복원된 이미지와 입력 이미지 사이의 MSE Loss를 Energy로 사용하였습니다.
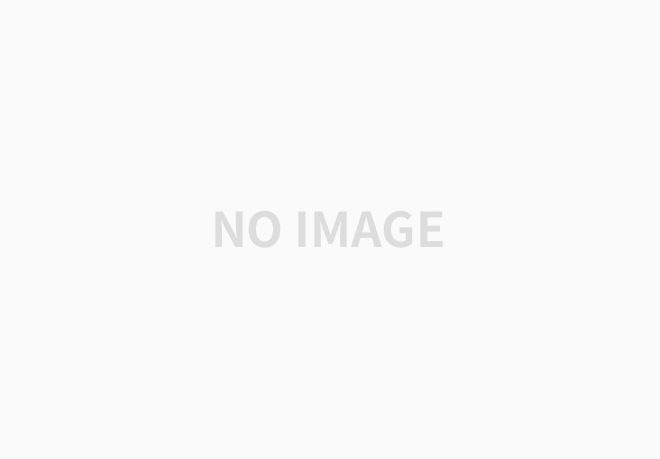
이제 다시 Score-based Model로 돌아와서 생각해봅시다. Score-based Model은 이 Energy에 대한 개념을 Loss가 아니라 확률 분포(Probability Distributions)에 대한 개념으로 생각해야 합니다.
pθ(x)=1Zθe−fθ(x)
fθ(x)는 neural network로 모델링된 energy based function이며 Zθ는 ∫pθ(x)dx=1이여야 하기 때문에 이를 위하기 위한 normalization 상수 입니다. Energy가 낮을 수록 p(x)의 값은 높아지기 때문에 위에서 생각한 energy의 개념과 같습니다.
다만 Maximum Likelihood 방법을 사용하기 위해서는 Zθ=∫e−fθ(x)dx인 상수를 쉽게 계산할 수 있어야 합니다. 그렇지만 딱히 방법이 없기 때문에 우리는 Zθ를 무시하고 근사할 수 있는 방법을 생각해야 합니다.
Score function을 근사한 신경망 sθ(x)에 대한 표현으로 나타내 봅시다.
∇xlogpθ(x)=∇xlog(1Zθe−fθ(x))=∇xlog1Zθ+∇xloge−fθ(x)=−∇xfθ(x)≈sθ(x)
위와같이 Normalization 상수를 포함하지 않고 표현이 가능합니다. GT score function에 대한 fisher divergence는 다음과 같습니다.
Ep(x)[||sθ(x)−∇logp(x)||22]
Fisher Divergence
Fisher Divergence는 확률 분포간 차이를 측정하는 방법 중 하나입니다. KL Divergence와 비슷한 개념이지만 KL Divergence는 비대칭적이고 Fisher Divergence는 대칭적인 성질을 가지고 있습니다. Fisher Divergence의 수식은 다음과 같습니다.
F(p,q)=12(Tr(Cov(q)−1Cov(p))+(μq−μp)TCov(q)−1(μq−μp)−k+lndet(Cov(q))det(Cov(p)))
Cov는 공분산 행렬, Tr은 행렬의 대각 요소의 합입니다. det는 determinant로 행렬식입니다. 즉 p와 q의 평균과 공분산을 이용해 두 분포사이의 거리를 측정합니다. Eq 157은 아마 이를 간단하게 표현한 것 같습니다.
우리는 모든 x에 대한 likelihood를 높임으로써 dataspace에서 어떤 방향으로 이동해야되는지를 학습합니다. score function은 dataspace에서 mode를 가리키는 vector field를 정의하는 역할을합니다. 말이 좀 어려운데 mode는 최빈값을 나타내고 (GAN의 단점이라 할 수 있는 mode collapse의 mode입니다.) vector field는 아래 그림을 보면 바로 이해가 될 것 같습니다.
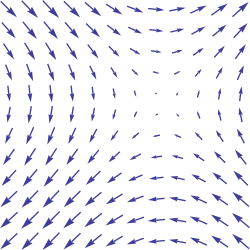
https://en.wikipedia.org/wiki/Vector_field
Vector field - Wikipedia
From Wikipedia, the free encyclopedia Assignment of a vector to each point in a subset of Euclidean space A portion of the vector field (sin y, sin x) In vector calculus and physics, a vector field is an assignment of a vector to each point in a space,
en.wikipedia.org
Vector field는 흐름의 크기와 방향을 나타낸 지도라고 생각할 수 있습니다. true data distribution의 score function을 학습하여 mode에 도달할 때까지 반복적으로 표본을 생성합니다.
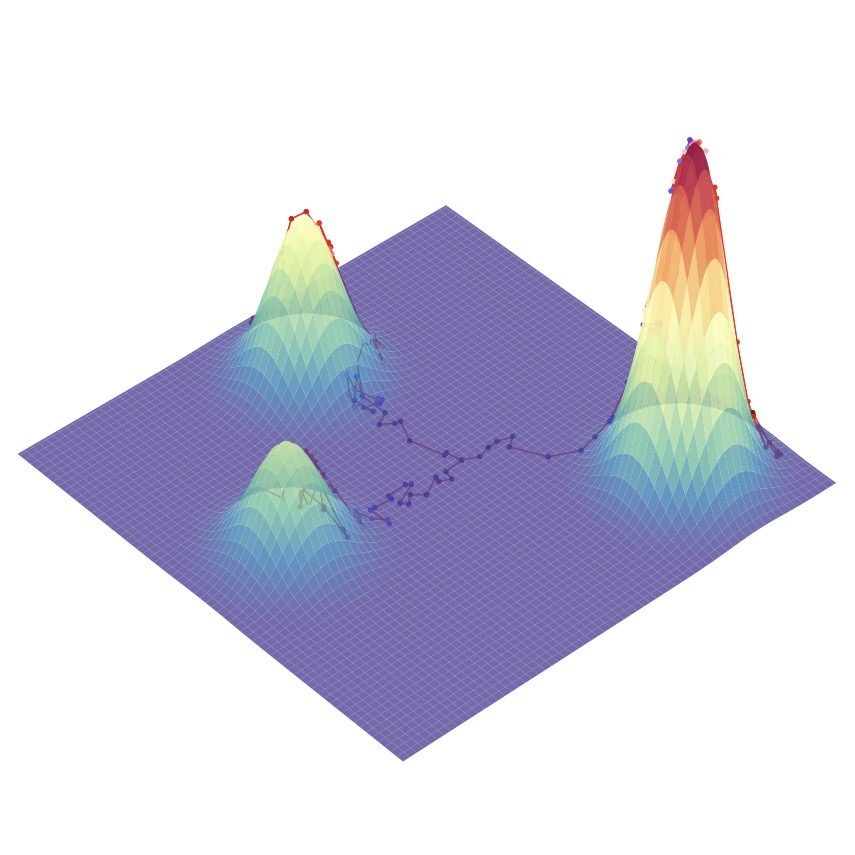
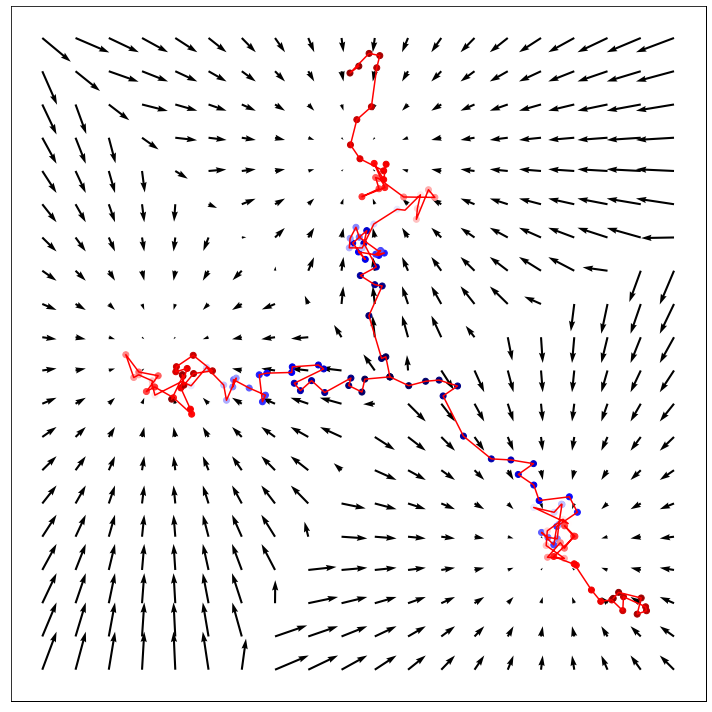
위의 그림은 score function을 통해 정의된 vector field를 따라 mode를 찾아가는 모습을 시각화 한 것입니다.
표본을 추출하는 프로세스는 Langevin dynamics를 사용합니다.
Langevin Dynamics (랑주벵 동역학)
Brownian motion(브라운 운동)은 입자들이 불규칙하게 운동하는 현상입니다. 여기서 Langevin Dynamics는 브라운 운동을 수학적으로 모델링한 미분 방정식(differential equation)입니다.
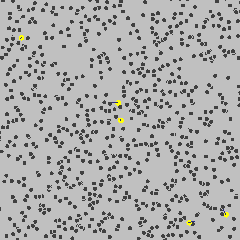
https://ko.wikipedia.org/wiki/%EB%B8%8C%EB%9D%BC%EC%9A%B4_%EC%9A%B4%EB%8F%99
브라운 운동 - 위키백과, 우리 모두의 백과사전
위키백과, 우리 모두의 백과사전. 브라운 운동(Brownian motion)은 1827년 스코틀랜드 식물학자 로버트 브라운(Robert Brown)이 발견한, 액체나 기체 속에서 미소입자들이 불규칙하게 운동하는 현상이다.
ko.wikipedia.org
https://en.wikipedia.org/wiki/Langevin_equation
Langevin equation - Wikipedia
From Wikipedia, the free encyclopedia Stochastic differential equation In physics, a Langevin equation (named after Paul Langevin) is a stochastic differential equation describing how a system evolves when subjected to a combination of deterministic and fl
en.wikipedia.org
브라운 운동에 대한 Langevin Dynamics는 다음과 같이 정리할 수 있습니다.
F=m¨x=−λ˙x+η
m은 입자의 속도이고 λ는 damping coefficient(감쇠 계수)이며 η∼N(0,2σ2)는 Gaussian noise입니다. (F는 Force 즉 힘입니다.)
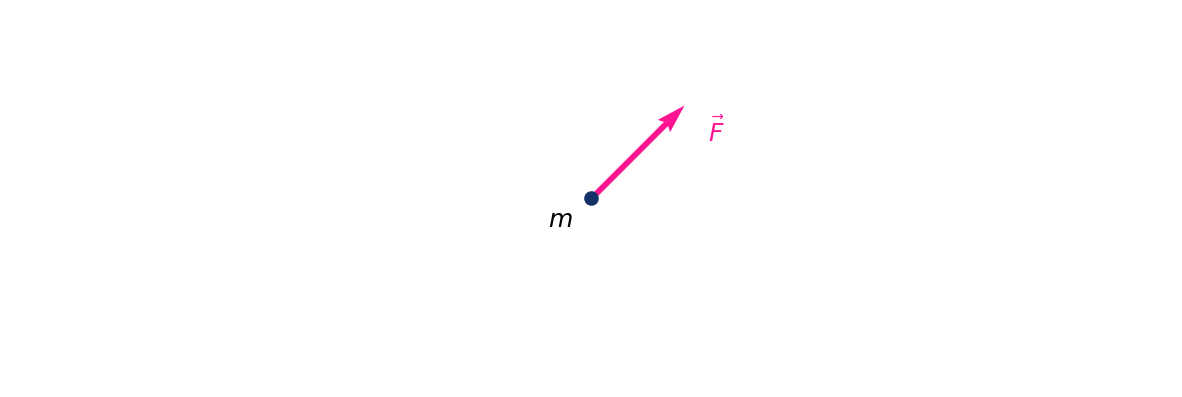
이때 물리에서 입자의 Force(F)는 Potential energy V(x)의 미분으로 나타낼 수 있습니다. 수식을 정리하면 아래와 같이 나타낼 수 있습니다.
m¨x=F=∂V(x)∂x=∇V(x)∇V(x)=−λ˙x+ηλ˙x=−∇V(x)+η
여기에 Stochastic Differenctial Equation (SDE)를 넣으면 다음과 같이 나타낼 수 있습니다.
dx=−∇V(x)dt⏟drift term+√2σdW⏟diffusion term
여기서 −∇V(x)는 drift term, σ는 Volatility(변동성), 그리고 dW는 표준 Weiner process의 시간 t에따른 도함수(미분)입니다. 음.. 자세히는 모르겠지만, 확실한건 σdw는 이 식의 불확실성을 의미하고 무작위의 diffusion에 대한 force를 의미한다는 것입니다. 다음 그림을 보시면 직관적으로 이해할 수 있습니다.
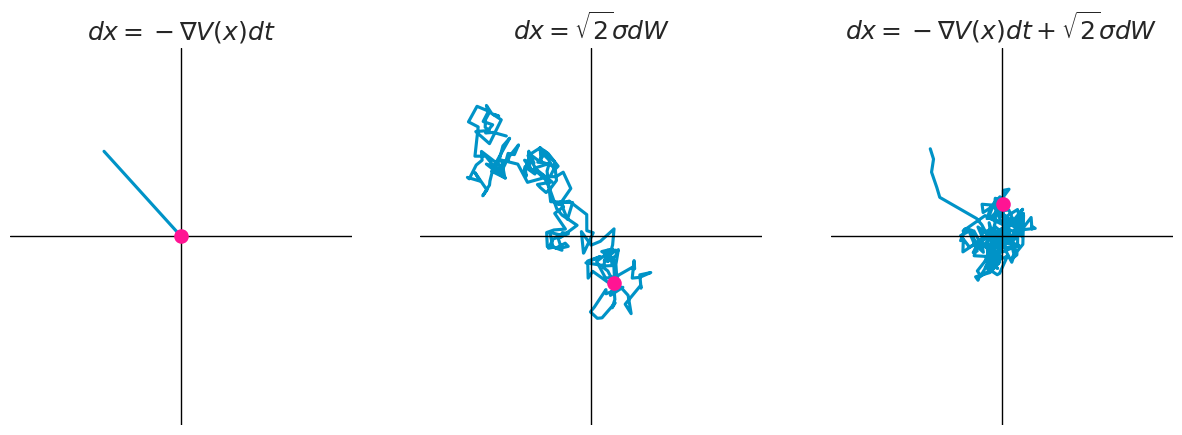
SDE는 따로 확률미분방정식에 대한 공부가 없다면 이해하기 힘들 수 있습니다. 아래 블로그에 잘 설명되어 있으니 혹시 더 정확한 수학적 설명을 원하시면 참고해 주세요.
확률미분방정식
freshrimpsushi.github.io
Steady State of Langevin Dynamics
Langevin dynamics을 통해 분포로부터 표본을 추출하기 위해서는 Langevin dynamics가 생성하는 분포(q(x,t))를 특정할 수 있어야 합니다. 이를 위해 시간에 따른 확률 밀도함수의 미분 방정식인 Fokker-Planck equation을 이용해봅시다.
먼저 Standard Wiener process W를 통해 구현된 Itô process(이토 확률 과정)의 가장 기본적인 SDE는 다음과 같습니다:
dx=μ(x,t)dt+σ(x,t)dW
앞서 나온 SDE에서는 여기에 −∇V(x)와 √2σ를 대입한 것입니다. 여기서도 마찬가지로 μ(x,t)는 drift term, σ(x,t)는 Volatility입니다.
여기서 우리는 D(x,t)=σ(x,t)2/2를 diffusuion coefficient(확산 계수)라고 정의하겠습니다. 이때 위의 SDE에 대한 Fokker-Plank equation은 다음과 같이 정의됩니다:
∂∂tp(x,t)=−∂∂x[μ(x,t)p(x,t)]+∂2∂x2[D(x,t)p(x,t)]
https://en.wikipedia.org/wiki/Fokker%E2%80%93Planck_equation
Fokker–Planck equation - Wikipedia
From Wikipedia, the free encyclopedia Partial differential equation A solution to the one-dimensional Fokker–Planck equation, with both the drift and the diffusion term. In this case the initial condition is a Dirac delta function centered away from zero
en.wikipedia.org
우리는 p(x,t)를 알 수 없기 때문에 q(x,t)를 통해 수식을 나타내야 합니다. p(x,t)=q(x,t)를 대입하고 마찬가지로 이전 SDE에서 대입한 μ(x,t)=∇V(x)와 σ(x,t)=√2σ를 통해 Langevin Dynamics의 식으로 고쳐봅시다.
∂∂tq(x,t)=∂∂x[∇V(x)q(x,t)]+∂2∂x2[σ2q(x,t)]
식이 유도되었습니다!! 위 식에서는 x와 t라는 변수가 있네요. 이 Fokker-Plank equation을 x와 t에 대해 시뮬레이션한 그래프를 그려보면 아래 그림과 같이 나타나게됩니다.
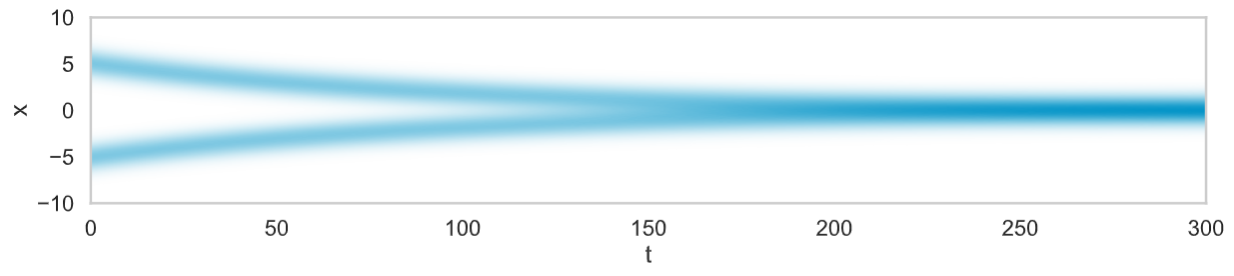
그림을 보면, t가 커짐에 따라 x가 0으로 수렴하게 됩니다. 이때 그래프의 기울기는 0이 될테니 ∂∂tq(x,t)=0이되겠죠? 이 때 limt→∞ q(x,t)=q∞(x)라 합시다. 그리고 아래와 같이 가정하고 식을 정리해봅시다.
limt→∞q(x,t)=q∞(x)∂∂tq∞(x)=∂∂x[∇V(x)q∞(x)+∂∂x[σ2q∞(x)]]=∂∂xJ=0
여기서 J는 Probability current(확률 흐름)이라고 하며, 임의의 공간 x에 대한 확률밀도의 흐름의 정도를 나타내는 값입니다. 그냥 [∇V(x)q∞(x)+∂∂x[σ2q∞(x)]]를 나타내는 정도로만 이해합시다. 어쨌든 우리는 위의 그래프에서 t가 무한대일 때, q∞(x)의 미분만 0에 수렴하는 것이 아니라 값 도 0에 수렴하는 것을 알 수 있습니다. 즉 모든 x에 대하여 J(x)=0입니다. 그렇다면 다음과 같이 식을 유도할 수 있습니다.
J(x)=[∇V(x)q∞(x)+∂∂x[σ2q∞(x)]]=0∂∂x[σ2q∞(x)]=−∇V(x)q∞(x)∂∂xq∞(x)=−∇V(x)σ2q∞(x)
q∞(x)를 미분했는데 q∞(x)가 있는 식이 나오네요. 그렇다면 q∞(x)는 ef(x)꼴의 함수라고 추측할 수 있습니다.
∂∂xef(x)=∇[f(x)]ef(x)이기 때문입니다. 그렇다면 q∞(x)에 대한 완전한 함수를 정의하는 것은 힘들더라도 비례식은 세울 수 있습니다 ∇V(x)는 V(x)의 미분이고, σ를 상수 취급했을 때 다음과 같은 비례식을 유도할 수 있습니다:
q∞(x)∝exp(−V(x)σ2)
위의 비례식은 Boltzmann distribution(혹은 Gibbs distribution)의 비례식과 같습니다.
https://en.wikipedia.org/wiki/Boltzmann_distribution
Boltzmann distribution - Wikipedia
From Wikipedia, the free encyclopedia Probability distribution of energy states of a system Boltzmann's distribution is an exponential distribution. Boltzmann factor p i p j {\displaystyle {\tfrac {p_{i}}{p_{j}}}} (vertical axis) as a function of temperatu
en.wikipedia.org
그렇다면 비례식에서 normalization constant인 Z를 통해 비례식이 아닌 방정식으로 나타낼 수 있습니다. Energy-based models의 form처럼 바꾸자면, 우리가 실제로 추정하는 p(x)에 대하여, p(x)=exp[−V(x)]Z 입니다. (V(x)=E(x)즉 Energy Function 이기 때문에 이같은 형태로 정의가 가능합니다.) 다음과 같이 정리할 수 있습니다.
p(x)=exp[−V(x)]Zlogp(x)=logexp[−V(x)]Zlogp(x)=log1Z+logexp[−V(x)]logp(x)−log1Z=logexp[−V(x)]V(x)=−logp(x)+log1Z∇V(x)=−∇logp(x)
Z는 상수이기 때문에 미분 시 0입니다. 앞서 제시한 SDE의 drift term에 대입하여봅시다.
dx=∇logp(x)dt+√2σ∇W
Sampling Using Langevin Dynamics
SDE를 실제 데이터의 분포(혹은 그 비슷한 것)라 가정했을 때, 이를 직접 추정하는 것은 굉장히 큰 Cost가 생깁니다. 따라서 우리는 효율적인 학습을 위해 이에 대한 추정치를 통해 값을 근사해야합니다. 즉 Euler-Maruyama 방법(Euler 방법)에 의해 SDE에 대한 근사 수치를 나타낼 수 있습니다.
dx=f(x,t)dt+g(x,t)dWyn+1=yn+f(y,n)Δt+g(y,n)ΔW
이때 Δt는 timestep에 대한 일정한 간격을 나타냅니다. timestep t가 [0, T]일 때, 간격은 Δt=T/N입니다. y는 x를 근사한 값으로 y0=x0입니다. 마지막을 ΔW는 평균 0, 표준편차 Δt인 정규분포를 따릅니다. (ΔW∼N(t;0,Δt)) 이 방법을 적용하면 다음과 같이 근사값을 나타낼 수 있습니다.
dx=∇logp(x)dt+√2σdWxn+1=xn+∇logp(xn)Δt+√2σΔW
여기서 결국 ΔW∼N(t;0,Δt)이므로 ΔW에 reparameterization trick을 적용할 수 있습니다.
z∼N(0,1)일 때
xn+1=xn+∇logp(xn)Δt+σ√2Δtz
우리는 이 식을 통해 SDE를 유도 할 수 있습니다. 아래는 Gaussian Distribution과 N(0,1), N(5,0.1)인 각 분포가 timestep에 따라 Euler-Maruyama 방법에 의해 유도되는 q(x,t)를 시각화 한 것입니다.
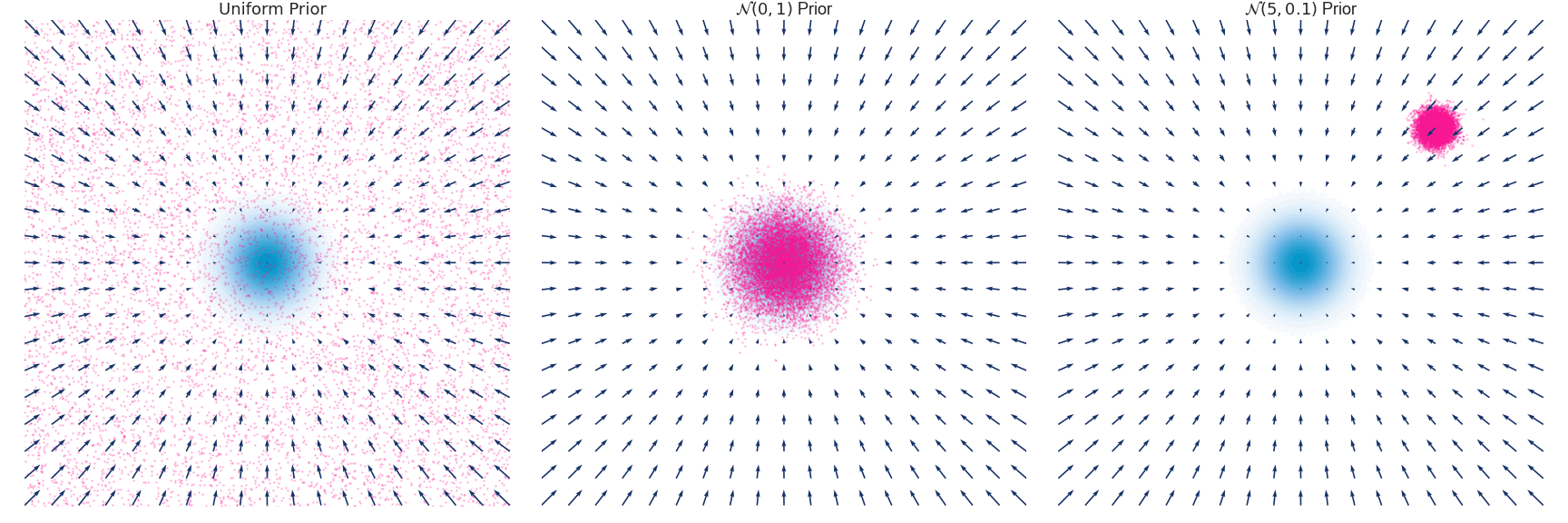
https://abdulfatir.com/blog/2020/Langevin-Monte-Carlo/
Monte Carlo Sampling using Langevin Dynamics | Abdul Fatir Ansari
Langevin Monte Carlo is a class of Markov Chain Monte Carlo (MCMC) algorithms that generate samples from a probability distribution of interest (denoted by π) by simulating the Langevin Equation. The Langevin Equation is given by \[\lambda\frac{dX_t}{d
abdulfatir.com
https://perceptron.blog/langevin-dynamics/
Langevin Monte Carlo: Sampling using Langevin Dynamics
A tutorial on how to sample from a distribution using Langevin Dynamics, why it works.
perceptron.blog
https://simpling.tistory.com/31
논문 리뷰: SDE-Net: Equipping Deep Neural Networks with Uncertainty Estimates
"SDE-Net: Equipping Deep Neural Networks with Uncertainty Estimates" (2020, ICML) - Lingkai Kong et al. https://en.wikipedia.org/wiki/Euler%E2%80%93Maruyama_method https://arxiv.org/abs/1806.07366 https://en.wikipedia.org/wiki/Euler_method https://github.c
simpling.tistory.com
이제 SDE를 유도하는 Langevin Dynamics를 우리의 식에 적용해봅시다. i=n, c=Δt, ϵ=z을 적용하면 Langevin Dynamics를 다음과 같이 나타낼 수 있습니다.
xi+1←xi+c∇logp(xi)+√2cϵ,i=0,1,…,K
x0는 real data의 분포에서 random하게 sampling한 값이고 ϵ∼N(ϵ;0,I)는 extra noise term으로 생성되는 sample들이 mode collapse되지 않도록 하면서 mode 주위를 맴돌도록 해주는 역할을 합니다. (즉 똑같은 이미지를 생성하지 않으면서도 mode(최빈값)에 가까울 수록 더 자연스러운 이미지일테니 이 주위를 맴도는 sample을 추출하도록 하는 역할입니다.) 또한 학습된 Score function은 Deterministic하기 때문에 생성 프로세스에 Stochastic한 값을 추가하여 이를 우회하는 의미도 있습니다. 예를 들면 아래 그림처럼 mode가 2개일 때 유용하게 작용할 수 있습니다.
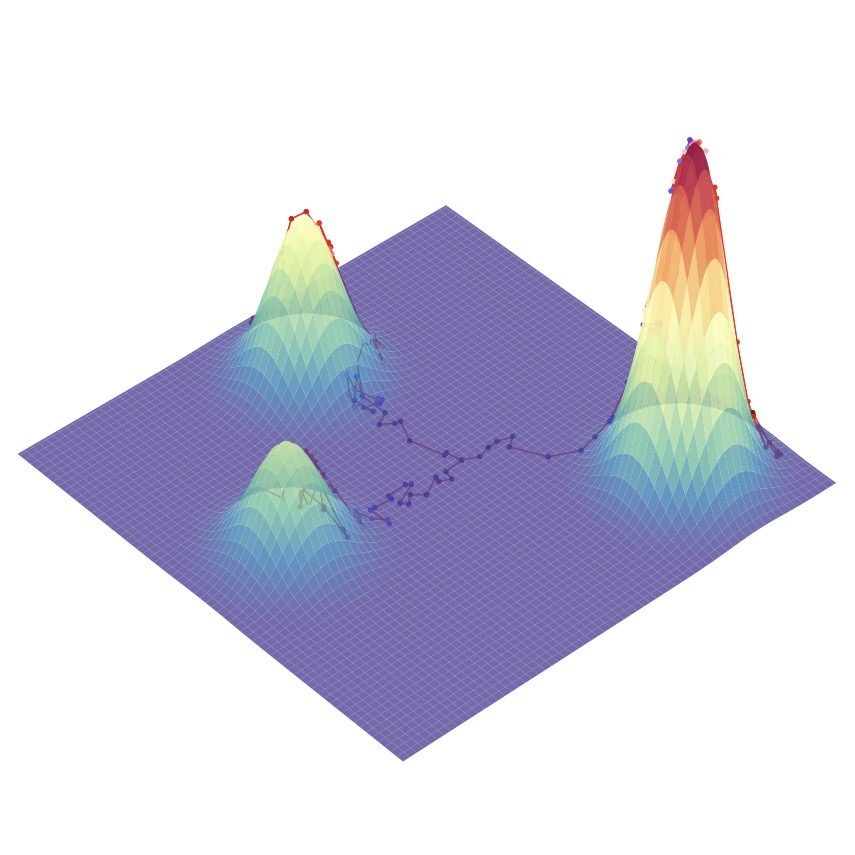
다만 여기서 ground truth score function은 우리가 예측하기 힘든 real data의 매우 복잡한 분포를 가지고 있습니다. 다행히도 우리는 score matching을 통해 ground truth score 없이도 Eq 157의 Fisher Divergence를 minimize 할 수 있습니다.
'Generative Models' 카테고리의 다른 글
생성 모델 논문 추천 List - Generative Adversarial Networks (2) | 2023.12.11 |
---|---|
[논문 리뷰] Understanding Diffusion Models: A Unified Perspective (2) (4) | 2023.08.12 |
[논문 리뷰] Understanding Diffusion Models: A Unified Perspective (1) (0) | 2023.07.06 |
[논문 리뷰] StyTr²: Image Style Transfer with Transformers (0) | 2023.06.07 |